Artificial intelligence (AI) has revolutionized the way we approach problem-solving, and Russian NN models have emerged as a powerful force in this domain. These models leverage advanced neural networks to deliver groundbreaking results across various industries. As AI continues to evolve, understanding Russian NN models becomes increasingly important for businesses and researchers alike.
Russian NN models have gained significant attention due to their exceptional performance in natural language processing (NLP) and computer vision tasks. These models are developed by top-tier Russian research institutions and tech companies, showcasing the country's expertise in AI and machine learning. With their ability to handle complex datasets and deliver accurate predictions, Russian NN models have become indispensable tools in the AI landscape.
This article delves deep into the world of Russian NN models, exploring their architecture, applications, and impact on global AI development. Whether you're a researcher, developer, or simply curious about AI, this guide will provide you with valuable insights into one of the most exciting areas of machine learning today.
Read also:Rudy Pankow At 13 The Remarkable Journey Of A Rising Star
Table of Contents
- Introduction to Russian NN Models
- Architectural Overview
- Applications of Russian NN Models
- Comparison with Global Standards
- Key Players in Russian AI
- Advantages and Challenges
- Real-World Impact
- Future Trends
- Ethical Considerations
- Conclusion
Introduction to Russian NN Models
Russian NN models represent a significant advancement in artificial intelligence, combining cutting-edge research with practical applications. These models are designed to tackle some of the most complex problems in machine learning, including language translation, image recognition, and predictive analytics. With their robust architecture and extensive training datasets, Russian NN models have become a benchmark for AI innovation.
One of the standout features of Russian NN models is their adaptability. These models can be fine-tuned for specific tasks, making them highly versatile across industries. From healthcare to finance, Russian NN models are being deployed to improve efficiency, accuracy, and decision-making processes. The ability to process large volumes of data in real-time further enhances their value in today's fast-paced digital world.
Furthermore, Russian NN models benefit from the country's strong tradition in mathematics and computer science. This foundation has enabled researchers to develop innovative solutions that push the boundaries of what is possible in AI. As a result, Russian NN models have gained recognition on the global stage, contributing to the advancement of AI as a whole.
Architectural Overview
The architecture of Russian NN models is designed to maximize performance and accuracy. These models typically employ deep learning techniques, utilizing multiple layers of neural networks to process and analyze data. The complexity of the architecture allows for the extraction of intricate patterns and relationships within datasets, leading to more accurate predictions and insights.
Neural Network Design
Russian NN models are built using advanced neural network architectures, such as transformers, recurrent neural networks (RNNs), and convolutional neural networks (CNNs). These architectures are specifically chosen based on the type of task the model is designed to perform. For example, transformers are commonly used for natural language processing tasks, while CNNs excel in image recognition.
- Transformers: Enable efficient processing of sequential data, such as text or speech.
- RNNs: Ideal for tasks involving temporal dependencies, such as time-series analysis.
- CNNs: Specialized for image and video processing, delivering high accuracy in computer vision tasks.
Training Methodologies
Training Russian NN models requires vast amounts of data and computational resources. Researchers employ a variety of training methodologies to ensure that the models achieve optimal performance. Techniques such as transfer learning, fine-tuning, and unsupervised learning are commonly used to enhance the capabilities of these models.
Read also:Panthers Fan Flash The Ultimate Guide For Diehard Panthers Supporters
Data augmentation is another critical aspect of training Russian NN models. By expanding the dataset through transformations and modifications, researchers can improve the model's ability to generalize and handle diverse inputs. This approach helps to mitigate overfitting and ensures that the model performs well in real-world scenarios.
Applications of Russian NN Models
Russian NN models have found applications across a wide range of industries, transforming the way businesses operate and delivering tangible benefits. Some of the key applications include:
- Healthcare: Enhancing diagnostic accuracy through medical imaging analysis and personalized treatment recommendations.
- Finance: Improving fraud detection, risk assessment, and algorithmic trading through predictive analytics.
- Retail: Optimizing inventory management and enhancing customer experience through personalized recommendations.
- Transportation: Advancing autonomous driving technology and improving traffic management systems.
These applications demonstrate the versatility and impact of Russian NN models in addressing real-world challenges. As the technology continues to evolve, new use cases are likely to emerge, further expanding the potential of these models.
Comparison with Global Standards
When compared to global standards, Russian NN models hold their own against some of the best AI systems in the world. While models developed in countries like the United States and China have garnered significant attention, Russian NN models offer unique advantages that set them apart.
One of the key differentiators is the focus on efficiency and scalability. Russian NN models are often designed with resource-constrained environments in mind, making them more accessible to organizations with limited computational capabilities. Additionally, the emphasis on interpretability ensures that these models can be trusted and understood by end-users.
However, challenges remain in terms of funding and international collaboration. To fully realize the potential of Russian NN models, greater investment and partnerships are needed to bridge the gap with global leaders in AI development.
Key Players in Russian AI
Several organizations and institutions have played a pivotal role in the development of Russian NN models. These include:
- Sberbank: A leading financial institution in Russia, Sberbank has invested heavily in AI research and development, producing several cutting-edge NN models.
- Yandex: Known for its search engine and AI-driven services, Yandex has developed numerous NN models that power its products and platforms.
- Skolkovo Institute of Science and Technology (Skoltech): A research university focused on innovation, Skoltech has contributed significantly to the advancement of Russian NN models through its cutting-edge research programs.
These organizations, along with others, have established Russia as a formidable player in the global AI landscape. Their commitment to innovation and collaboration continues to drive the development of Russian NN models forward.
Advantages and Challenges
Russian NN models offer numerous advantages, but they also face challenges that must be addressed to ensure their continued success.
Competitive Advantages
Some of the key advantages of Russian NN models include:
- Efficiency: Designed for resource-constrained environments, these models can operate effectively even with limited computational resources.
- Interpretability: Emphasis on transparency ensures that users can understand and trust the decisions made by these models.
- Specialization: Russian NN models are often tailored to specific tasks, delivering superior performance in niche applications.
Overcoming Challenges
Despite their advantages, Russian NN models face challenges such as:
- Funding: Limited financial resources can hinder the development and deployment of these models on a larger scale.
- Global Recognition: Greater efforts are needed to promote Russian NN models on the international stage and attract collaborations with global partners.
Addressing these challenges will be crucial for the long-term success of Russian NN models in the global AI market.
Real-World Impact
The impact of Russian NN models extends beyond theoretical advancements, delivering tangible benefits in real-world applications. For example, in the healthcare sector, these models have improved diagnostic accuracy, leading to better patient outcomes. In finance, they have enhanced risk assessment and fraud detection, helping organizations mitigate potential losses.
Moreover, Russian NN models have contributed to the development of autonomous systems, such as self-driving cars and drones, revolutionizing the transportation industry. The ability to process vast amounts of data in real-time has enabled these systems to operate safely and efficiently, paving the way for a new era of mobility.
Future Trends
As AI continues to evolve, the future of Russian NN models looks promising. Emerging trends such as federated learning, edge computing, and quantum computing are likely to play a significant role in shaping the next generation of these models.
Federated learning, for instance, allows models to be trained on decentralized data, ensuring privacy and security while maintaining performance. Edge computing enables real-time processing at the source of data generation, reducing latency and improving efficiency. Quantum computing, while still in its infancy, holds the potential to revolutionize AI by solving problems that are currently beyond the reach of classical computers.
Ethical Considerations
With great power comes great responsibility. As Russian NN models become more advanced, ethical considerations must be addressed to ensure their responsible use. Issues such as bias, transparency, and accountability must be carefully managed to prevent unintended consequences.
Researchers and developers must prioritize fairness and inclusivity in the design and training of these models. Regular audits and evaluations should be conducted to identify and mitigate biases that may arise during the development process. Additionally, clear guidelines and regulations should be established to govern the deployment and use of Russian NN models in various applications.
Conclusion
Russian NN models represent a significant advancement in artificial intelligence, offering unparalleled capabilities in natural language processing, computer vision, and predictive analytics. Through their innovative architecture and practical applications, these models have made a lasting impact on industries worldwide.
As the field of AI continues to evolve, Russian NN models are poised to play a crucial role in shaping the future of technology. By addressing current challenges and embracing emerging trends, these models can achieve even greater heights in the years to come.
We invite you to join the conversation by leaving your thoughts and questions in the comments section below. Share this article with your network and explore other resources on our site to deepen your understanding of AI and machine learning. Together, let's unlock the full potential of Russian NN models and drive innovation forward.
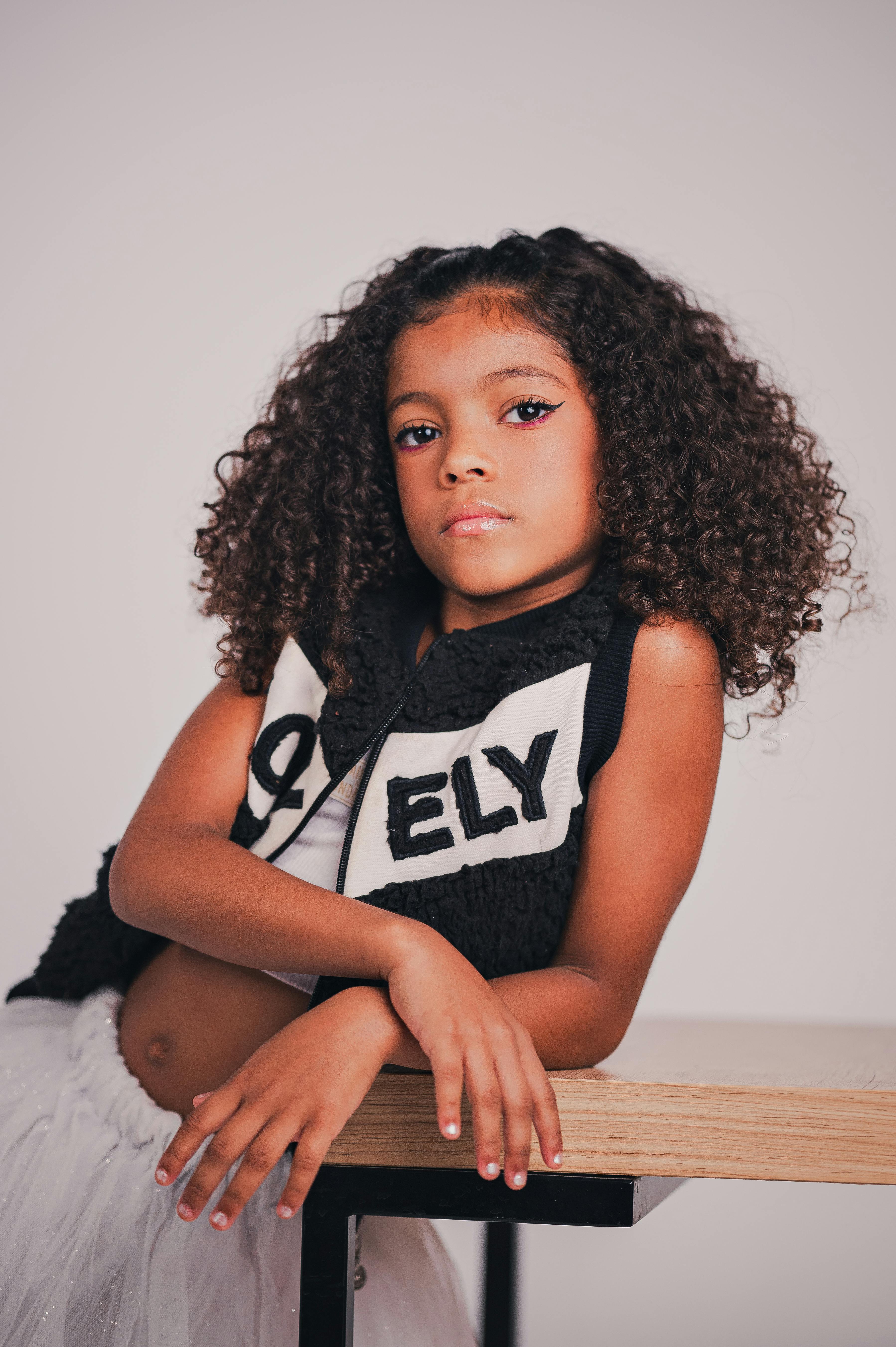

